Single-cell phenotypes of peripheral blood immune cells in early and late stages of non-alcoholic fatty liver disease
Article information
Abstract
Background/Aims
Immune and inflammatory cells respond to multiple pathological hits in the development of nonalcoholic steatohepatitis (NASH) and fibrosis. Relatively little is known about how their type and function change through the non-alcoholic fatty liver disease (NAFLD) spectrum. Here we used multi-dimensional mass cytometry and a tailored bioinformatic approach to study circulating immune cells sampled from healthy individuals and people with NAFLD.
Methods
Cytometry by time of flight using 36 metal-conjugated antibodies was applied to peripheral blood mononuclear cells (PBMCs) from biopsy-proven NASH fibrosis (late disease), steatosis (early disease), and healthy patients. Supervised and unsupervised analyses were used, findings confirmed, and mechanisms assessed using independent healthy and disease PBMC samples.
Results
Of 36 PBMC clusters, 21 changed between controls and disease samples. Significant differences were observed between diseases stages with changes in T cells and myeloid cells throughout disease and B cell changes in late stages. Semi-supervised gating and re-clustering showed that disease stages were associated with fewer monocytes with active signalling and more inactive NK cells; B and T cells bearing activation markers were reduced in late stages, while B cells bearing co-stimulatory molecules were increased. Functionally, disease states were associated with fewer activated mucosal-associated invariant T cells and reduced toll-like receptor-mediated cytokine production in late disease.
Conclusions
A range of innate and adaptive immune changes begin early in NAFLD, and disease stages are associated with a functionally less active phenotype compared to controls. Further study of the immune response in NAFLD spectrum may give insight into mechanisms of disease with potential clinical application.
Graphical Abstract
INTRODUCTION
Non-alcoholic fatty liver disease (NAFLD) is the most common cause of chronic liver disease worldwide. NAFLD affects approximately 25% of the Western population [1] and represents a spectrum of liver disease, including steatosis, non-alcoholic steatohepatitis (NASH), which can lead to fibrosis, and in some patients, cirrhosis, liver failure, and liver cancer [2,3]. Currently, NASH can only be distinguished from steatosis using liver biopsy, to grade and stage histological features of liver injury, inflammation, and fibrosis. Immune and inflammatory cell infiltration, together with hepatocyte injury, are the pathological hallmarks of non-alcoholic steatohepatitis (NASH). However, little is known about the composition and functional status of circulating immune and inflammatory cells in patients with NASH, particularly in the pre-cirrhotic stages. This is despite the fact that peripheral blood can be readily sampled and may give diagnostic and therapeutic insights into disease—two areas in which clinical progress is limited by our understanding of the mechanisms that drive NASH [4,5].
Current dogma is that a background of metabolic co-morbidity, genetic, dietary, and environmental factors predispose to multiple pathogenic hits that determine progression through the NAFLD spectrum. These hits include lipid-mediated hepatocyte injury through oxidative stress, effects of obesogenic diets on intestinal epithelial function and the microbiome which together activate damage-signalling pathways, and innate inflammatory responses [6]. Lipid accumulation is believed to trigger oxidative stress in hepatocytes, generating damage associated molecular patterns that are sensed by the toll-like receptor (TLR) family. TLRs also detect gut-derived pathogen associated molecular patterns,such as lipoteichoic acid, lipopolysaccharide, and flagellin (ligands for TLRs 2, 4, and 5, respectively). TLR binding activates intracellular transcription factors including ERK, MAP kinases, and nuclear factor kappa B (NF-κB) and, indirectly, JAK-STAT proteins. Together, these release cytokines and chemokines drive inflammatory and fibrogenic processes and further compound metabolic dysfunction.
To date, a limited number of studies have shown that peripheral blood pro-inflammatory Th1 cells are increased in patients with NASH [3,4]; a phenotype also observed in people with obesity, who additionally have increased Th17 cells and reduced Th2 and regulatory T cells [7-9]. Natural killer (NK) cells sampled from patients with NASH express higher levels of activation markers NKG2D, CD25, and CD69, and can produce higher levels of inflammatory cytokinesin response to ex vivo stimulation compared to controls [10]. Phenotypic shifts in monocytes have been described in people with NAFLD compared to controls, such as increase in intermediate and non-classical subsets and expression of cell surface TLRs, CD169, and CCR4 [5,11-13]. Fibrosis risk is increased in patients with hepatic steatosis and detectable autoantibodies or raised serum immunoglobulins [14], but the role of B cells that produce these antibodies has yet to be explored. Thus, emerging data indicate that lipid-related inflammation in the liver has the potential to activate immune responses, and we hypothesise that this leads to changes in immune cell phenotype and function.
So far, studies of immune cell populations have largely depended on fluorescence-based techniques which, until recently, were limited by the number of cell types or functions that can be assessed in a single sample. Mass cytometry allows large numbers of markers to be assessed and analysed simultaneously at a single-cell level. Traditional gating-based approaches restrict analysis to known or pre-defined cell types, limiting the discovery of novel immune functions. Therefore, bioinformatic approaches are needed to combine prior knowledge with unsupervised high-dimensional clustering to interpret mass cytometry data where multiple features are examined together.
We hypothesised that the peripheral composition and phenotype of peripheral blood immune cells differed between healthy controls, patients with steatosis, and those with NASH. We used single-cell cytometry by time of flight (CyTOF), together with a tailored unsupervised and semi-supervised bioinformatic pipeline, to report changes in total peripheral blood mononuclear cells (PBMCs) and at high resolution in T, B, and NK cells and monocyte-containing populations at early (steatosis) and late stages (NASH) of the NAFLD spectrum.
MATERIALS AND METHODS
Patients and samples
Patients and healthy volunteers were recruited from Barts Health NHS Trust and Queen Mary, University of London. Study protocols were approved by the East London and City Regional Ethics Committee (reference number 14/WA/1142) and performed in compliance with the Declaration of Helsinki. All participants gave their written informed consent. We included adult patients (age >18 years) with evidence of liver steatosis based on imaging (ultrasound, computed tomography, magnetic resonance imaging) or histology. Patients were excluded if they had any coexisting chronic liver disease diagnoses other than NAFLD, consumed alcohol greater than 14 units per week, or had clinical features of decompensated cirrhosis. Transient elastography was performed to measure liver stiffness according to standard clinical practice (reliable liver stiffness result based on successful reading rate >60% and interquartile range of all readings <30% of the median). Liver biopsy was performed in selected patients when clinically indicated. Each liver biopsy was reported by a single histopathologist (in routine clinical care) and were summarised according to the National Institutes of Health NASH clinical research network (Kleiner) criteria [15].
PBMCs were sampled from 64 individuals (21 healthy, 43 with different stages of NAFLD), of which 19 samples (three healthy, 16 with NAFLD) were analysed by CyTOF. Ex vivo assays and conventional flow cytometry were used to confirm and extend CyTOF findings in the other 45 individuals (10 healthy, 35 with NAFLD). For CyTOF, those with NAFLD were subcategorised into steatosis (n=6) and NASH with fibrosis (n=10) according to liver histology or non-invasive fibrosis scores (Supplementary Table 1), giving three groups referred to as control,steatosis, and NASH, respectively.
Cell separation
PBMCs were separated by density gradient over Ficoll-Paque (GE Healthcare, Uppsala, Sweden).
Mass cytometry
We designed a panel of 36 metal-conjugated antibodies targeted against cell surface and intracellular proteins,selected to enable phenotyping and functional characterization (activation of intracellular signalling pathways, including the Toll-like receptor pathways, JAK-STAT signalling and NF-κB activation) of major immune cell subsets (https://www.biolegend.com/en-us/cell-markers) (Supplementary Table 2). Antibodies were either obtained pre-conjugated (Fluidigm, San Fransisco, CA, USA) or conjugated in-house with trivalent metal isotopes using the MaxPAR antibody conjugation kit (Fluidigm). To select the most optimal metal isotope and antibody clone combination, the Maxpar Panel Designer tool was used (https://www.fluidigm.com/products-services/technologies/mass-cytometry) (Supplementary Table 2). One million PBMCs were washed with cell staining buffer (Fluidigm) before being incubated for 5 minutes at room temperature with Fc block (Biolegend, Munich, Germany). Surface marker antibodies were added for 30 minutes at room temperature. Cells were washed with cell staining buffer and fixed with 2% paraformaldehyde (PFA) (Sigma-Aldrich, St. Louis, MO, USA) for 10 minutes, followed by two further washes in cell staining buffer, and then permeabilised with 90% methanol for 30 minutes on ice. Cells were washed once with phosphate-buffered saline (PBS) and once with cell staining buffer, and then stained with intracellular antibodies for 45 minutes at room temperature. Cells were washed with FoxP3 permeabilisation buffer (eBioscience, San Diego, CA, USA) and stained with FoxP3 for 30 minutes at room temperature. Cell-ID Intercalator-Ir was diluted with Fix and Perm Buffer (Fluidigm) to 100 nM, added to each tube, and then left overnight or for a maximum of 3 days at 4˚C. Cells were washed twice with ultrapure water (Milli-Q; Millipore Corporation, Bedford, MA, USA), added to an aqueous suspension of normalization beads (Fluidigm), and filtered through a 35-μm membrane prior to mass cytometry analysis.
Flow cytometry
Surface staining was performed on cells washed with FACS buffer (PBS, 2% FCS, 0.05% sodium azide, 0.5 mM EDTA). Dead cells were detected with Zombie NIR™ Fixable Viability Kit (Biolegend) according to the manufacturer’s protocol. Cells were then stained for 20 minutes at room temperature with antibody mixes including the following: CD3-PeCy7, CD3-PerCPCy5.5, CD4-FITC, CD4-PECy7, CD8-PerCPCy5.5, CD14-PerCPCy5.5, CD16-FITC, CD45RO-PE, CD45RA-APC, CCR6-PE, CXCR3-APC, and HLA-DR-PECy7 (all from Biolegend). For intracellular staining, cells were washed after surface staining, fixed with 2% PFA for 15 minutes at RT, and washed with permeabilization buffer (FoxP3 eBioscience buffer). Cells were then intracellular stained for 30 minutes at RT with antibody mixes that were made in permeabilization buffer, including interferon (IFN)γ-PB and IL-4-AF647 (all from Biolegend). Cells were washed with FACS buffer and acquired using a BD Canto II. All experiments were analysed using FlowJo v10.4 (Becton, Dickinson and Company, Ashland, OR, USA).
CyTOF bioinformatic analysis
All bioinformatics software package versions used in this pipeline are outlined in Supplementary Table 3.
Pre-processing, batch correction, and dimension reduction
Normalised (Fluidigm, normalisation passport EQ-P13H2302 version 2) live single-cell cisplatin-negative FCS files were exported from Cytobank [16] and converted into CSV format using the ‘flowCore’ package. Median marker intensities of all live cisplatin negative cells were transformed using the hyperbolic sine transform (arcsinh) with a cofactor of 1. We subsampled an equal number of 500,000 cells from each condition (healthy, steatosis, and NASH) and pooled these into a single data frame comprising 1.5 million cells by 36 marker dimensions. Cells were then batch corrected for variation using the CyCombine method [17]; in summary, we used an 8×8 grid and apply the ‘scale’ normalisation approach. Dimension reduction was carried out the batch the corrected transformed cells using the Uniform Manifold Approximation and Projection (UMAP) algorithm [18]. UMAP parameters were set to 15 nearest neighbours and a minimum distance of 0.1.
Immunophenotypic cell subset identification
Phenotyping by accelerated refined community-partitioning (PARC) [19] was applied to carry out unsupervised identification of immunophenotypic cell subsets on all batch-corrected cells. Default PARC parameters were used. Re-clustering of manually gated populations were carried out for semi-supervised high-resolution analysis; in these instances, the ‘small_pop’ parameter of PARC was set to 5,000 cells. Cluster abundances across patients were analysed via principal component analysis. Input to the principal component analysis was the abundance of each cluster for each patient. Cluster abundances were z-score normalised and visualised using the ‘heatmap.2’ package in R to visualise the abundance of each immunophenotypic cluster for each patient.
Statistical analysis
The diffcyt pipeline [20] was used to carry out differential immunophenotypic cluster abundance analysis (diffcyt-DA-edgeR) in R using the previously identified PARC clusters. A design matrix was created to conduct three comparisons: NASH relative to control, NAFLD relative to NASH, and NASH relative to NAFLD. Cluster counts were normalised using weighted trimmed mean of M-values to account for composition effects of cluster counts across patient groups. Each contrast resulted in an output table (produced using the top Table function) with summary statistics on the log2 fold change of the normalised cluster abundances and Benjamini-Hochberg adjusted P-values to account for multiple testing [21]. A controlled false discovery rate (FDR) level of <0.05 was considered statistically significant.
Data visualisation
Bar plots and cluster-marker bubble diagrams were generated using the ‘ggplot2’ package in R. Hierarchical clustering was carried out using the ‘ggdendro’ package in R.
RESULTS
NAFLD disease stage characterised by changes in peripheral immune cell phenotype
Compared to the steatosis group, the median age of the NASH group was older (61 vs. 50 years, P=0.03) with higher prevalence of type 2 diabetes (70% vs. 17%, P<0.0001). The median body mass indices in steatosis and NASH groups were significantly higher compared to the control group (31.0 kg/m2 and 28.9 kg/m2, respectively, compared to 23.2 kg/m2, P=0.004).
Immune cell cluster distribution differed between the control, steatosis, and NASH groups (Fig. 1A, B). Principal component analysis showed separation of the control individuals away from NASH (Fig. 1C), with steatosis samples lying in an intermediate position.

(A) PARC live cell clusters visualised on a two-dimensional UMAP plot. UMAP plots show changes in PARC clusters at the single cell level in the control, steatosis, and NASH patients. In the combined UMAP, grey represents single cells in steatosis and NASH that overlap with control. Yellow represents unique cells in steatosis compared to control, and red represents unique cells in NASH compared to control. An equal number (500,000) of cells from each group (control, steatosis, NASH) have been subsampled. (B) Heatmap representing z-score normalised cluster abundance across all patient groups and all live cell clusters. Rows represent the z-normalised cluster abundance across all clusters for each patient, columns patient expression profiles across clusters. Columns are coloured by either green (control patients), blue (steatosis patients), and pink (NASH patients). (C) PCA plot based on cluster abundance across patient groups. Green circles represent control patients (n=3), blue circles represent steatosis patients (n=6), and red circles represents NASH patients (n=10). PARC, phenotyping by accelerated refined community-partitioning; UMAP, Uniform Manifold Approximation and Projection; NASH, non-alcoholic steatohepatitis; PCA, principal components analysis.
To study known cell types in different stages of disease, we applied a pre-defined classical gating strategy (Supplementary Fig. 1) to the mass cytometry-acquired single cell dataset. We found significant differences in the proportions of live cells of Th1, Th2, cytotoxic T cells, and myeloid-derived suppressor cells (Supplementary Fig. 2). We also confirmed the changes in Th1 and Th2 cells in an independent group of samples from fifteen patients with NASH and fibrosis and eight healthy controls using flow cytometry (Supplementary Fig. 3, Supplementary Table 1).
To gain detailed insight into the changes in immune cells in different stages of disease, we applied unsupervised methods to cluster cells according to relative expression (Fig. 2A circle size) and median intensity (Fig. 2A circle colour) of each antibody signal. Twenty-three out of 36 clusters changed significantly in abundance between disease states. Four clusters (0, 13, 20, and 30) increased in steatosis and NASH groups compared to the control group (Fig. 2B). A further four clusters (9, 25, 26, and 34) increased in NASH group compared to steatosis group. Conversely, six clusters (4, 16, 19, 21, 23, and 27) had fewer cells in NASH group compared to steatosis group. Some changes were only statistically significant when comparing NASH group with the control group (17, 24, and 28 increased; 1, 11, 14, 15, 18, and 29 decreased).
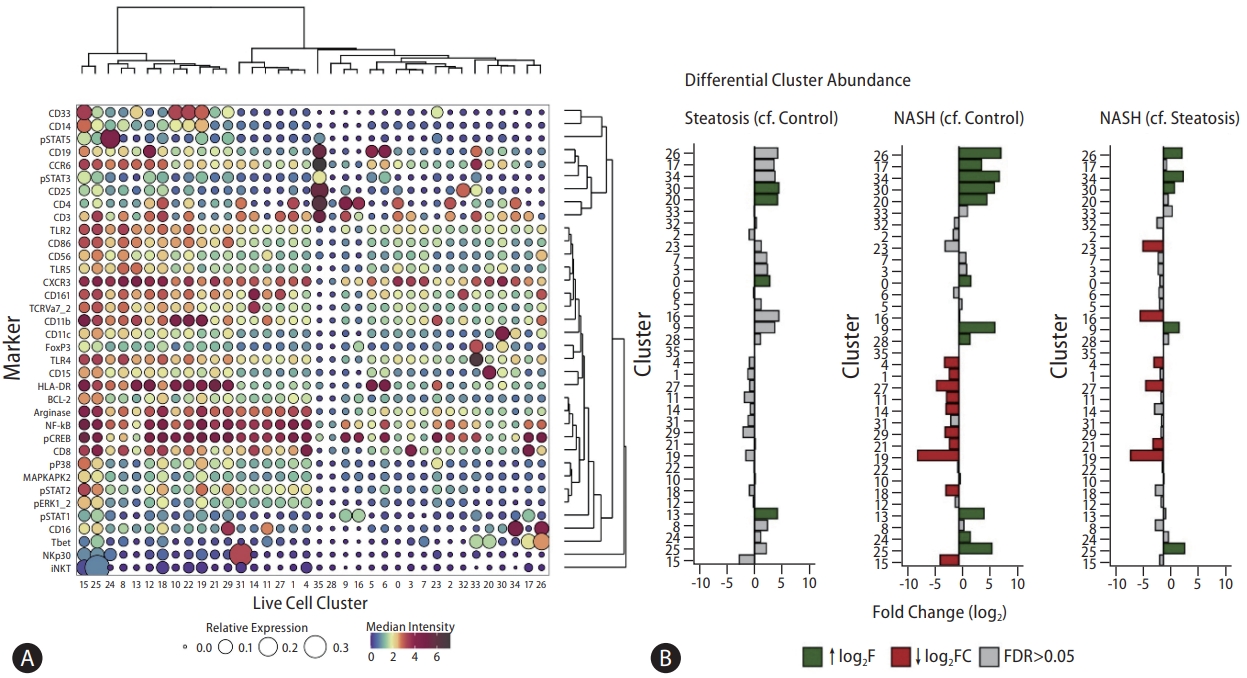
(A) Balloon plot representing relative and median intensity profiles across live cell clusters for all markers. The size of each circle represents the relative expression of each marker across all clusters. Each circle is coloured based on the median intensity of each marker in a given cluster. Markers with shared expression profiles across clusters are located together (right dendrogram) and clustering of cells with shared median marker expression profiles across markers are located together (top dendrogram). Dark brown circles represent high relative expression and high median intensities. (B) Differential cluster abundance analysis between control, steatosis, and NASH patients. A bar plot to show the log2 fold change in cluster abundance. Log2 fold change refers to the change in cluster abundance between steatosis patients compared to control patients, NASH patients compared to control patients, and NASH patients compared to steatosis patients, respectively. Coloured bars represent clusters that reach statistical significance (FDR <0.05). Grey bars represent clusters that do not reach statistical significance (FDR >0.05). NASH, non-alcoholic steatohepatitis; FDR, false discovery rate.
Number and function of known cell types change in NAFLD
Based on the pattern of marker expression, 14 clusters could be assigned to known existing cell types: seven bore T cell markers, four myeloid, two NK cell, and one mucosal-associated invariant T (MAIT) cell markers. Among the clusters that expressed myeloid markers, cluster 13 (increased in steatosis) expressed HLA-DR, TLR2, and TLR5 strongly, but had lower levels of CD14, phosphorylated (p)NF-κB, pCREB, and arginase. Cluster 19 (reduced in NASH) also expressed HLADR strongly and had higher levels of pNF-κB, pCREB, arginase, pSTAT2, and CD14, with low levels of CD16. Cluster 14 cells expressed CD3, CD161, and TCRVa7.2 with high levels of pNF-κB and pCREB, indicating that the cluster contained transcriptionally active MAIT cells and was significantly less abundant in NASH group compared to steatosis and control groups.
Of the seven T cell groups, two clusters (4 and 17) that expressed high-intensity CD8 (marker of cytotoxic T cells) and three clusters (0, 9, and 34) that expressed high-intensity CD3, CD4, and CXCR3 (Th1 markers) (Fig. 2B) increased in disease states, which was consistent with the findings of the manual gating of mass cytometry and flow cytometry (Supplementary Figs. 2, 3). We identified Cxcr3+ Ifng+ Th1 cells in a search of the Liver Cell Atlas(https://www.livercellatlas.org/datasets_NAFLDmouse.php) [22], derived from single-cell analysis of Western diet-fed mouse liver tissue (Supplementary Figs. 4). We hypothesised that lipid-mediated hepatocyte injury could drive a ‘skew’ in T helper cell differentiation towards a Th1 phenotype. To test this, we incubated hepatocyte-like HepG2 cells with a 2:1 combination of palmitic acid and oleic acid (1 mM and 0.5 mM) for 24 hours to model lipid-induced hepatocyte injury. Naïve T cellsisolated from healthy controls were then incubated in media supplemented with supernatant from the fatty-acid treated HepG2 cells. This resulted in a higher proportion of CD3+CD4+CXCR3+ cells (Supplementary Fig. 5A) and higher expression levels of the Th1 cytokine IFNγ (Supplementary Fig. 5B) compared to the naïve T cells cultured in supernatant from control-treated HepG2 cells. Taken together, these data indicate that lipid-mediated hepatotoxicity generates a milieu that can induce naïve T cells to differentiate towards Th1 cells as identified by CyTOF.
Collectively, our data demonstrate that shifts in broad immune cell types occur in both steatosis and NASH. However, this combination of traditional analysis and unsupervised clustering does not make use of the granularity of the large number of markers included in the panel, leaving nine clusters uncharacterised. A more detailed study is needed to understand the functional status of all cell types that are changing in number. Therefore, in order to more fully utilise this high dimensional dataset and to gain new insights into immune cell subsets, we applied high-dimensional single-cell unsupervised analytical techniques to broad gates of known cell groupings: CD3+CD19- (includes T cells), CD19+CD3- (includes B cells), CD3-CD19-CD14-CD56+ (includes NK cells), and CD3-CD19-CD14+ (includes monocytes); and re-clustered cells within each group. These broad groups accounted for 89% of total cells.
Changes in CD3+ T cell clusters throughout the NAFLD spectrum
The abundance of eight out of 18 clusters of CD3+ cells (Fig. 3A, B) changed between the control, steatosis, and NASH groups(Fig. 3B, D). The greatest number of cluster differences was observed between NASH group and controls; although many of these changes were apparent in steatosis group versus controls, they did not reach statistical significance. CD3+ cluster 13 (Fig. 3C) expressed the highest levels of CD161 and TCRVa7.2 (MAIT cell markers); and, consistent with the unsupervised analysis, was lower in patients with NASH compared to the controls. We confirmed this reduction in activated MAIT cells in the blood sampled from an independent group of 14 patients with NASH and fibrosis or steatosis and seven healthy controls using classical fluorescence flow cytometry (Supplementary Figs. 6).
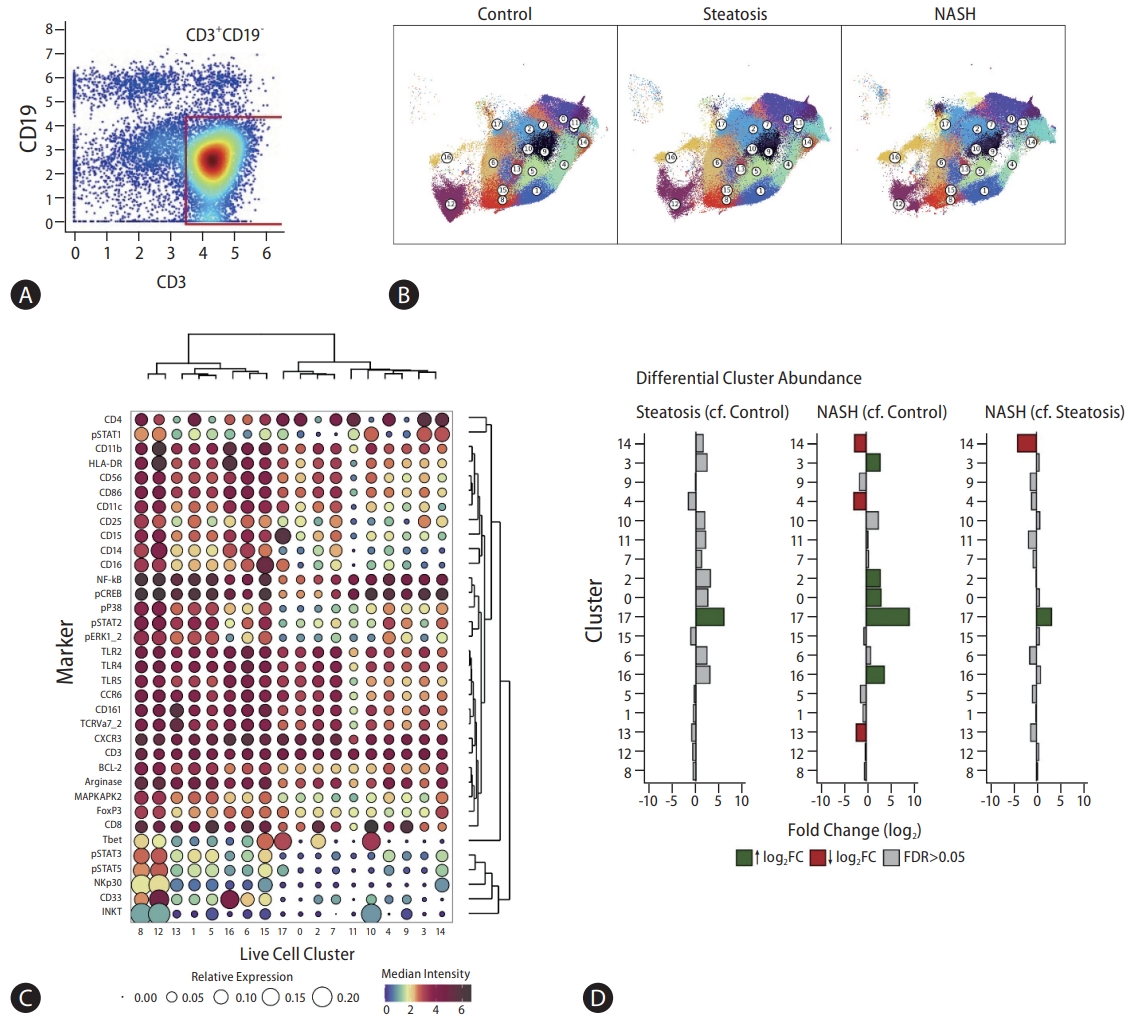
(A) CD3+CD19- gating strategy. (B) CD3+CD19- clusters visualised on a two-dimensional UMAP plot. UMAP plots show changes in CD3+CD19- single cell clusters between control, steatosis, and NASH patients. Each colour represents a single cluster. Circle labels indicate cluster numbers. (C) Balloon plot representing relative and median intensity profiles across CD3+CD19- clusters for all markers. The size of each circle represents the relative expression of each marker across all clusters. Each circle is coloured based on the median intensity of each marker in a given cluster. Markers with shared expression profiles across clusters are located together (right dendrogram), and clustering of cells with shared median marker expression profiles across markers are located together (top dendrogram). (D) Differential CD3+CD19- cluster abundance analysis between control, steatosis, and NASH patients. A bar plot to show the log2 fold change in cluster abundance. Log2 fold change refers to the change in cluster abundance between steatosis patients compared to control patients, NASH patients compared to control patients, and NASH patients compared to steatosis patients, respectively. Coloured bars represent clusters that reach statistical significance (FDR <0.05). Grey bars represent clusters that do not reach statistical significance (FDR >0.05). UMAP, Uniform Manifold Approximation and Projection; NASH, non-alcoholic steatohepatitis; FDR, false discovery rate.
Expressions of CD4 and CD8 were largely reciprocal across clusters (Fig. 3C). Disease state-related changes were observed in five CD4-expressing (0, 3, 4, 14, and 17) and three CD8-expressing (2, 13, and 16) clusters. CD4-expressing cluster 17, characterised by high levels of CD4 and T-bet, increased in both steatosis and NASH comparisons. Since this cluster included CXCR3- and CCR6-expressing cells, it may contain different groups of T helper cell, although these cells may be less active with lower levels pNF-κB, pERK1&2, and pSTATs. In keeping with this increase in cells with low signalling activity, CD4-expressing clusters (4 and 14) that co-expressed pP38-, pSTAT2-, and pERK1&2 reduced in abundance. Similarly, CD8-expressing clusters 2 and 16 that increased were characterised by less intense signalling molecules, including MAPKAP, BCL2, pP38, pSTAT2 (but not other STATs), and pERK1&2, compared to cluster 13, which reduced in abundance. Taken together, both the activation status of T cells as well as their phenotype should be taken into account when distinguishing between NASH, steatosis, and healthy patients.
Changes in CD3-CD19+ B cells in patients with steatosis and NASH compared to controls
Compared to controls, four clusters of CD3-CD19+ cells (Fig. 4A, B) changed in abundance in NASH group, but not in steatosis (Fig. 4B, D). All CD3-CD19+ clusters also expressed high intensity of HLA-DR indicative of B cells (Fig. 4C). Clusters 0 and 8 that increased were characterised by higher levels of the following: co-stimulatory molecule CD86; memory markers CD11b, CXCR3, CD11c, CD33; and TLRs 2, 5, and to a lesser extent, TLR4. Clusters that decreased in abundance expressed higher levels of pCREB and pERK1&2, which are related to the activation status. In the mouse Liver Cell Atlas [22], CD33 was principally expressed in neutrophils, plasmacytoid dendritic cells, and monocyte derived cells, but not in B cells (Supplementary Figs. 7).

(A) CD3-CD19+ gating strategy. (B) CD3-CD19+ clusters visualised on a two-dimensional UMAP plot. UMAP plots show changes in CD3-CD19+ single cell clusters between control, steatosis, and NASH patients. Each colour represents a single cluster. Circle labels indicate cluster numbers. (C) Balloon plot representing relative and median intensity profiles across CD3-CD19+clusters for all markers. The size of each circle represents the relative expression of each marker across all clusters. Each circle is coloured based on the median intensity of each marker in a given cluster. Markers with shared expression profiles across clusters are located together (right dendrogram), and clustering of cells with shared median marker expression profiles across markers are located together (top dendrogram). (D) Differential CD3-CD19+ cluster abundance analysis between control, steatosis, and NASH patients. A bar plot to show the log2 fold change in cluster abundance. Log2 fold change refers to the change in cluster abundance between steatosis patients compared to control patients, NASH patients compared to control patients, and NASH patients compared to steatosis patients, respectively. Coloured bars represent clusters that reach statistical significance (FDR <0.05). Grey bars represent clusters that do not reach statistical significance (FDR >0.05). UMAP, Uniform Manifold Approximation and Projection; NASH, non-alcoholic steatohepatitis; FDR, false discovery rate.
Changes in CD3-CD19-CD14-CD56+ NK cells in steatosis and NASH
The abundance of five out of 11 clusters of CD3-CD19-CD14-CD56+ cells changed between control, steatosis, and NASH groups (Fig. 5). Clusters 0 and 8 were increased in steatosis group compared to the control group, and cluster 6 increased in NASH group compared to steatosis group—all three characterised by low expression of the activation marker NKp30. Reduced numbers of cells were observed in clusters 1 (steatosis) and 9 (NASH); both characterised by higher expression of phosphorylated signalling mediators: pERK, pCREB, pP38, and pSTATs 1, 2, 3, and 5. This suggests that, as with T cells, more advanced stages of NAFLD are associated with higher numbers of less active NK cells.
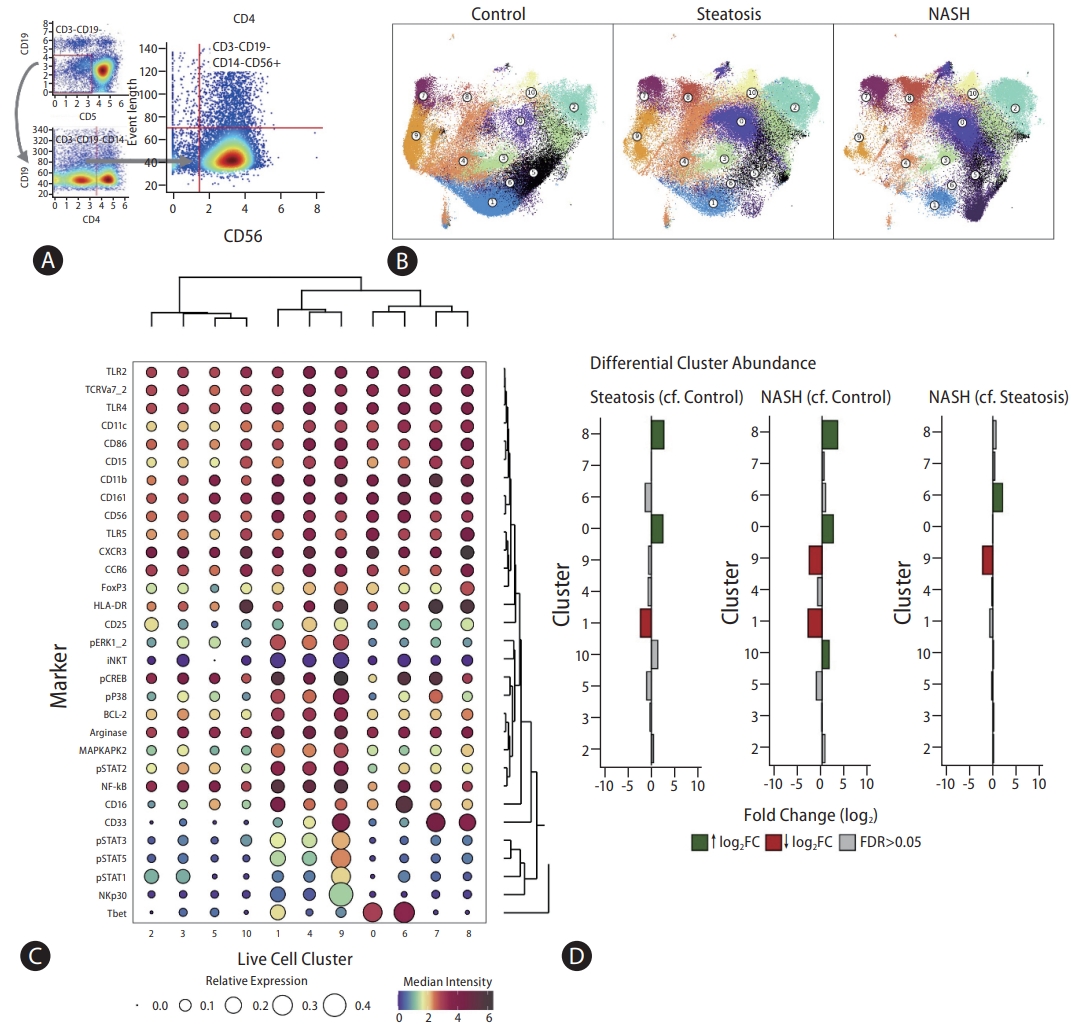
(A) CD3-CD19-CD14-CD56+ gating strategy. (B) CD3-CD19-CD14-CD56+ clusters visualised on a two-dimensional UMAP plot. UMAP plots show changes in CD3-CD19-CD14-CD56+ single cell clusters between control, steatosis, and NASH patients. Each colour represents a single cluster. Circle labels indicate cluster numbers. (C) Balloon plot representing relative and median intensity profiles across CD3-CD19-CD14-CD56+ clusters for all markers. The size of each circle represents the relative expression of each marker across all clusters. Each circle is coloured based on the median intensity of each marker in a given cluster. Markers with shared expression profiles across clusters are located together (right dendrogram), and clustering of cells with shared median marker expression profiles across markers are located together (top dendrogram). (D) Differential CD3-CD19-CD14-CD56+ cluster abundance analysis between control, steatosis, and NASH patients. A bar plot to show the log2 fold change in cluster abundance. Log2 fold change refers to the change in cluster abundance between steatosis patients compared to control patients, NASH patients compared to control patients, and NASH patients compared to steatosis patients, respectively. Coloured bars represent clusters that reach statistical significance (FDR <0.05). Grey bars represent clusters that do not reach statistical significance (FDR >0.05).
Changes in CD3-CD19-CD14+ cell clusters in patients with steatosis compared to controls
We identified nine clusters of CD3-CD19-CD14+ cells(Fig. 6A, C), four of which (expressing high levels of HLA-DR) changed in abundance between control, steatosis, and NASH groups (Fig. 6B, D). We also observed variation in the expression levels of CD14 and CD16 between the clusters that changed significantly, which went beyond the definitions of classical, intermediate, and non-classical subtypes. Cluster 6 was more abundant in NASH group compared to controls, and was characterised by less phosphorylated pSTAT2, MAPKAP2, pP38, pNF-κB, and pCREB staining. Cluster 4 (reduced in steatosis) and clusters 2 and 5 (reduced in NASH) were characterised by high expression and intensity of signalling mediators and TLRs (TLR2, TLR4, and TLR5). These findings are in keeping with, and give greater detail to, the observations in the unsupervised analysis, and suggest that NASH is associated with circulating monocytes that are less active and perhaps less responsive to TLR-mediated stimulation. To test this hypothesis, we stimulated peripheral blood mononuclear cells isolated from healthy individuals or n=9 validation group patients with NASH and fibrosis with lipopolysaccharide (LPS) or flagellin. LPS- and flagellin-induced interleukin (IL)-6 and tumor necrosis factor (TNF)α production were significantly impaired in NASH and fibrosis patients compared to controls, as was NF-κB phosphorylation (Fig. 6E-H).

(A) CD3-CD19-CD14+ gating strategy. (B) CD3-CD19-CD14+ clusters visualised on a two-dimensional UMAP plot. UMAP plots show changes in single cell CD3-CD19-CD14+ clusters between control, steatosis, and NASH patients. Each colour represents a single cluster. Circle labels indicate cluster numbers. (C) Balloon plot representing relative and median intensity profiles across CD3-CD19-CD14+ clusters for all markers. The size of each circle represents the relative expression of each marker across all clusters. Each circle is coloured based on the median intensity of each marker in a given cluster. Markers with shared expression profiles across clusters are located together (right dendrogram), and clustering of cells with shared median marker expression profiles across markers are located together (top dendrogram). (D) Differential CD3-CD19-CD14+ cluster abundance analysis between control, steatosis, and NASH patients. A bar plot to show the log2 fold change in cluster abundance. Log2 fold change refers to the change in cluster abundance between steatosis patients compared to control patients, NASH patients compared to control patients, and NASH patients compared to steatosis patients, respectively. Coloured bars represent clusters that reach statistical significance (FDR <0.05). Grey bars represent clusters that do not reach statistical significance (FDR >0.05). (E) Flow cytometry analysis of phosphorylated NFкB. PBMCs sampled from patients with NASH with fibrosis and healthy controls were stimulated with medium, LPS (1 μg/ mL) or flagellin (1 μg/mL), for 15 minutes and analysed by flow cytometry. (F) IL-6 production, (G) TNFα production, and (H) IL-10 production measured by ELISA. PBMCs sampled from patients with NASH with fibrosis and healthy controls were stimulated with medium, LPS (20 ng/mL) or flagellin (100 ng/mL), for 24 h before supernatants were collected for ELISA.
DISCUSSION
We used single-cell mass-cytometry together with a tailored unsupervised and supervised bioinformatics pipeline to detect a significant variation in innate and adaptive immune cell populations in the peripheral blood of patients with NAFLD. Patients and controls clustered separately, as well as semi-supervised high-resolution clustering showed phenotypic and functional heterogeneity within the known cell types. The main differences between steatosis and control groups were increases in NK and T cells with low levels of phosphorylated transcription factors. The majority of differences were observed between NASH and steatosis; an increase in B cells expressing memory markers and co-stimulatory molecules and further increase in NK and T cells with low levels of phosphorylated transcription factors paralleled by increase in NK, T, MAIT and B cells and monocytes with high levels of phosphorylated transcription factors. Functionally, monocytes taken from patients with more advanced stages of disease were less responsive to TLR agonists LPS and flagellin, in keeping with the single-cell data. Lipid-mediated hepatotoxicity in vitro generated a milieu that induced healthy naïve T cells to differentiate towards a Th1 phenotype, in keeping with our CyTOF and flow cytometry data in patients with steatosis and NASH and with murine data from the Liver Cell Atlas. Further mechanistic study is needed to confirm the drivers of Th1 differentiation in vivo.
Increasing evidence points towards a role for adaptive immunity in NASH pathogenesis. The inflammatory infiltrate in NASH contains B and T lymphocyte aggregates, and the size and number of these aggregates are correlated with the degree of inflammation and fibrosis [23]. Patients with obesity with or without type 2 diabetes, who are at the highest risk of NAFLD, have increased numbers of circulating Th1 and Th17 cells and higher levels of Th1-promoting IFNγ with a reciprocal reduction in Th2 and regulatory T cells. Although few have studied peripheral immune cells in people with simple steatosis, numbers of naive IFNγ-expressing CD4+ T cells were increased in the peripheral blood of 20 patients with NASH [7], and high levels of IFNγ mRNA expression in CD4+ T cells were observed in 51 patients with biopsy-proven NASH [8]. Consistent with this, we found that Th1 cells and CD8-expressing cytotoxic T cells were increased in patients with steatosis, and these remained elevated in NASH and fibrosis patients, albeit with lower levels of key phosphorylated signalling mediators, which may reflect an exhausted phenotype in NASH. This was consistent with recent findings of a novel subset of CXCR6+ CD8+ T cells with high expressions of both activation and exhaustion markers in NASH patients, which induced hepatocyte killing in an MHC-class-1-independent fashion via IL-15 and acetate in the liver [24]. Haas et al. [25] reported increase in activated cytotoxic CD8c T cells in NASH, which accumulate in close proximity to steatotic and injured hepatocytes in the liver. They also reported that circulating and liver CD8+ T cells correlated strongly with histological hallmarks of NASH: ballooning and lobular inflammation. Together, our study adds to the literature which shows that CD8+ T cells play a role in mediating hepatic inflammation and hepatocyte cell death in NASH, independently of antigen recognition.
Despite this evidence of antigen-independent T cell activation, specific antigens that could elicit an adaptive immune response have been identified in obesity and in NASH. Oxidised phospholipids and aldehydes form antigenic adducts called oxidised stress epitopes, antibodies against which can be detected in approximately 40% of patients with NAFLD or NASH [26]. The cellular infiltrate in NASH includes B lymphocytes [27], and selected depletion of B2 cells in mice results in mild NASH and less fibrosis [28]. B cell-derived inflammatory mediators activate hepatic stellate cells and the reciprocal production of retinoic acid can promote B cell maturation into plasma cells [29]. In NASH, we found increased B cells expressing CD33 along with co-stimulatory molecules,suggesting a role for antibody-producing B cells. However, it remains to be determined whether these B cells are clonal and whether a specific antibody-mediated response drives NASH. CD33 is a transmembrane receptor that is typically highly expressed on myeloid-committed cells, implicated in regulating cellular expansion, activation, and pro-inflammatory cytokine secretion [30]. Increased circulating CD33+ B cells have been reported in other systemic inflammatory conditions, including Behcet’s disease and sepsis [31], although CD33+ B cells were not identified in the murine Liver Cell Atlas. Further study is required to determine the pathogenesis of liver infiltrating B cell subsets in NASH.
We identified progressive changes in circulating MAIT cells; non-conventional innate-like T cells that express invariant T cell receptor (TCR) α-chain, composed of Va7.2-Ja33 and are restricted by the major histocompatibility complex class I-related molecule MR1 [32]. The current literature reports both protective and pathogenic roles in NASH [33,34]. We observed reduced circulating MAIT cells frequency in NASH group compared to steatosis and control groups, extending previous observations in patients with high NAFLD activity scores [33]. Similarly, reduced numbers of circulating MAIT cells accompanied by increased intra-adipose MAIT cell numbers have been reported in diabetes and obesity [35].
A recent study reported no significant change in the numbers of NK cells in patients with NAFLD, although there was an increase in NK cell activation marker NKG2D in patients with NASH and fibrosis [10]. Other studies have found reduced CD56dim NK cell subset with increased exhaustion markers, including programmed death 1 and immunoglobulin-like transcript 2, in NAFLD patients compared to the controls [36-38]. In the current study, although we did not have direct markers of exhaustion in our CyTOF panel, we found significant heterogeneity among NK cells with a reduction in the number of intracellular signalling mediators, including pERK, pCREB, pP38, and pSTATs 1, 2, 3, and 5, reflecting a less activated phenotype in NASH. Three clusters changed in abundance in steatosis, persisting through to NASH, and a further two clusters changed in NASH compared to steatosis. Given the emerging role of NK cells in modulating metabolic function [39,40] and the known associations of NK cells with liver disease in viral hepatitis [12,44,45], more detailed study of this heterogenous cells type is warranted.
Large numbers of myeloid cells reside in and patrol the liver, including Kupffer cells and blood-derived macrophages and dendritic cells. Recent single cell techniques have shown 14 subtypes of intrahepatic myeloid cells in advanced liver disease, including NASH [44]. We found nine clusters of circulating CD14+ cell, of which four changed in abundance in disease. These changes went beyond the traditional classification of monocytes into three groups based on CD14 and CD16 expression and intensity. Therefore, to delineate monocyte differentiation in NASH, further subclassification with other cell markers may be required [12,44,45]. Clusters with markers of activation were less abundant in patients with NASH and ex vivo, PBMCs sampled from patients with NASH produced lower amounts of TLR-mediated TNFα, IL-6, and IL-10 compared to the controls. This was similar to the findings in decompensated NASH cirrhosis [12], suggesting that changes in monocyte immunocompetence may begin earlier than those previously thought in NASH.
Our unsupervised and semi-supervised clustering methods address some of the limitations of traditional bi-axial gating approaches that require prior knowledge of immune cell populations. A significant advantage of using PARC as a clustering method was that we did not need to down-sample cells that could lead to information loss. The changes we observed by mass-cytometry were confirmed in independent samples analysed by fluorescence-based techniques, and the findings reported in earlier studies have been extended here. However, our study had a number of limitations. The cross-sectional nature of the study precludes causal conclusions to be drawn from our findings. People with NASH in this study were older and more obese than the individuals in the control group. Finally, a larger sample size would enable us to determine whether the changes we detect are related to the development of NASH or to metabolic inflammation observed in all patients with, for example, type 2 diabetes or obesity or early NASH, prior to the onset of liver fibrosis. We do not know the origin or mechanistic role of the cells detected in the pathogenesis of NASH. Our study focused on the peripheral immune compartment, and larger studies are needed to incorporate immune cells from the liver and adipose tissue combined with the approach and analytics used here to determine clonal and phenotypic relationships across tissue compartments. Whether the number and composition of immune cells can be used as non-invasive markers of disease stage or of response to treatment remains to be seen. However, better understanding of the function of the cells we have identified may lead to the discovery of soluble mediators that are more easily detected, which can be used for such clinical applications.
In conclusion, our study has demonstrated that innate and adaptive immune changes occur early in NAFLD and can be detected in the peripheral blood. We have used a tailored analytical pipeline and found heterogeneity among these cell types with distinct functional profiles. The role of the immune response in NAFLD warrants further attention.
Notes
Authors’ contribution
K.J.W., H.S., W.L. contributed equally to the manuscript. Data acquisition and analysis: K.J.W., H.S., W.L., J.H.B. Initial drafting of manuscript: K.J.W., W.L. Critical review and adaptation of the manuscript: A.D., C.B., W.K.S. and W.A. Study conception and supervision: W.A. and C.B. All authors approved the final version. Data, analytic methods, and study materials can be requested through corresponding author.
Conflicts of Interest
WA has received honoraria for speaking and consultancy from Gilead Sciences, Glaxosmithkline, Intercept and Coherus, and competitive funding from Gilead Sciences and Glaxosmithkline. He is supported by grant funding from the Medical Research Council. Other authors have no conflict of interest to declare.
Supplementary materials
Supplementary material is available at Clinical and Molecular Hepatology website (http://www.e-cmh.org).
NAFLD patient clinical characteristics
Panel of 36 antibodies used to characterise and phenotype all major immune cell subsets for CyTOF
Bioinformatic tools and software versions used in data analysis pipeline
Schematic depicts antibodies necessary to identify the cells of interest for CyTOF. Illustration shows antibodies required to detect peripheral immune cells starting from PBMCs in a sequential format. Positive markers are indicated by green arrow, and negative markers are indicated by red arrow.
PBMCs from patients with NASH and fibrosis have higher proportions of pro-inflammatory T helper 1 cells and cytotoxic T cells compared to controls. Populations of PBMCs from all controls, patients with steatosis, and patients with NASH, identified by pre-defined classical gating strategy to CyTOF-acquired single cell dataset using FlowJo.
Increased proportion of Th1 cells from PBMCs sampled from patients with NASH and fibrosis compared to healthy controls was confirmed by fluorescence flow cytometry. Proportions of Th1 cells were increased and Th2 cells were decreased in PBMCs sampled from patients with NASH and fibrosis compared to healthy controls when measured by flow cytometry. Proportions of Th17 cells did not differ between samples. No differences in cytotoxic T cells or MDSCs were observed between healthy controls and patients.
Presence of Cxcr3+ Ifng+ Th1 cells (cluster 7) in the liver from Liver Cell Atlas (https://www.livercellatlas.org/datasets_ NAFLDmouse.php). Plots were derived from a mouse model of NASH through cellular indexing of transcriptomes and epitopes by sequencing (CITEseq) analysis of immune cells isolated from the livers of mice fed on Western diet.
Naïve T cells isolated from healthy controls were incubated in media supplemented with supernatant from the fatty-acid treated HepG2 cells, resulting in increased Th1 cell skew. (A) Representative gating strategy to identify the proportion of CXCR3+CCR6- T helper cells, pre-gated on live, singlet CD3+CD4+ leukocytes. (B) Representative gating strategy to identify the proportion of IFNy+IL4- T helper cells, pre-gated on live, singlet CD3+CD4+ leukocytes. P values were determined by Man-Whitney U test (experiments n=3).
Reduction in activated MAIT cells in patients with NASH compared to controls using classical fluorescence flow cytometry. Representative gating strategy to identify the proportion of live, singlet CD3+ leukocytes that are CD161+TCRVα7.2+.
Presence of Cd33+ cells in the liver from Liver Cell Atlas (https://www.livercellatlas.org/datasets_NAFLDmouse. php). Plots were derived from a mouse model of NASH through cellular indexing of transcriptomes and epitopes by sequencing (CITEseq) analysis of immune cells isolated from the livers of mice fed on Western diet.
Abbreviations
CyTOF
cytometry by time of flight
FDR
false discovery rate
IFN
interferon
IL
interleukin
ILT2
immunoglobulin-like transcript 2
LPS
lipopolysaccharide
MAIT
mucosal-associated invariant T
MDSC
and myeloid-derived suppressor cell
NAFLD
non-alcoholic fatty liver disease
NASH
non-alcoholic steatohepatitis
NF- κB
nuclear factor kappa B
NK
natural killer
PARC
phenotyping by accelerated refined community-partitioning
PBMC
peripheral blood mononuclear cell
PBS
phosphate-buffered saline
PFA
paraformaldehyde
RT
FULL NAME
TCR
T cell receptor
TLR
toll-like receptor
TMM
trimmed mean of M-value
TNF
tumor necrosis factor
UMAP
Uniform Manifold Approximation and Projection
References
Article information Continued
Notes
Study Highlights
∙ In this study, we studied the differences between blood immune cells in people with different stages of non-alcoholic fatty liver disease.
∙ We used cytometry by time of flight (CyTOF) with bioinformatics analysis to detect previously uncharacterizable changes in peripheral immune cells in early and late stages of non-alcoholic fatty liver disease.
∙ We found that blood immune cells, particularly T and B cells, NK cells, and monocytes change in NASH and become less active. The pathogenic role of immune cells in this condition warrants further attention.